Invited Talks
There has recently been widespread discussion of whether GPT-3, LaMDA 2, and related large language models might be sentient. Should we take this idea seriously? I will discuss the underlying issue and will break down the strongest reasons for and against.
David Chalmers
David Chalmers is University Professor of Philosophy and Neural Science and co-director of the Center for Mind, Brain, and Consciousness at New York University. He is the author of THE CONSCIOUS MIND (1996), CONSTRUCTING THE WORLD (2010), and REALITY+ (2022). He is current president of the American Philosophical Association (Eastern Division). He co-founded the Association for the Scientific Study of Consciousness and the PhilPapers Foundation. He has given the John Locke Lectures and has been awarded the Jean Nicod Prize. He is known for formulating the “hard problem” of consciousness, which inspired Tom Stoppard’s play The Hard Problem; for the idea of the “extended mind,” which says that the tools we use can become parts of our minds; and for influential work on language and learning in neural network models and on other foundational issues in AI.
Conformal inference methods are becoming all the rage in academia and industry alike. In a nutshell, these methods deliver exact prediction intervals for future observations without making any distributional assumption whatsoever other than having iid, and more generally, exchangeable data. This talk will review the basic principles underlying conformal inference and survey some major contributions that have occurred in the last 2-3 years or. We will discuss enhanced conformity scores applicable to quantitative as well as categorical labels. We will also survey novel methods which deal with situations, where the distribution of observations can shift drastically — think of finance or economics where market behavior can change over time in response to new legislation or major world events, or public health where changes occur because of geography and/or policies. All along, we shall illustrate the methods with examples including the prediction of election results or COVID19-case trajectories.
Emmanuel Candes
Among the great challenges posed to democracy today is the use of technology, data, and automated systems in ways that threaten the rights of the American public. Too often, these tools are used to limit our opportunities and prevent our access to critical resources or services. These problems are well documented. In America and around the world, systems supposed to help with patient care have proven unsafe, ineffective, or biased. Algorithms used in hiring and credit decisions have been found to reflect and reproduce existing unwanted inequities or embed new harmful bias and discrimination. Unchecked social media data collection has been used to threaten people’s opportunities, undermine their privacy, or pervasively track their activity—often without their knowledge or consent.
These outcomes are deeply harmful—but they are not inevitable. Automated systems have brought about extraordinary benefits, from technology that helps farmers grow food more efficiently and computers that predict storm paths, to algorithms that can identify diseases in patients. These tools now drive important decisions across sectors, while data is helping to revolutionize global industries. Fueled by the power of American innovation, these tools hold the potential to redefine every part of our society and make life better for everyone.
This important progress must not come at the price of civil rights or democratic values, foundational American principles that President Biden has affirmed as a cornerstone of his Administration. To advance President Biden’s vision, the White House Office of Science and Technology Policy has identified five principles that should guide the design, use, and deployment of automated systems to protect the American public in the age of artificial intelligence.
The Blueprint for an AI Bill of Rights is a guide for a society that protects all people from these threats—and uses technologies in ways that reinforce our highest values. Responding to the experiences of the American public, and informed by insights from researchers, technologists, advocates, journalists, and policymakers, this framework is accompanied by From Principles to Practice—a handbook for anyone seeking to incorporate these protections into policy and practice, including detailed steps toward actualizing these principles in the technological design process. These principles help provide guidance whenever automated systems can meaningfully impact the public’s rights, opportunities, or access to critical needs.
Alondra Nelson
Alondra Nelson, Ph.D., (NAM) is the Harold F. Linder Professor at the Institute for Advanced Study. She currently serves as Deputy Assistant to the President and Deputy Director for Science and Society in the White House Office of Science and Technology Policy, where she performed the duties of the Director from February to October 2022. Dr. Nelson is most widely known for her research at the intersection of science, technology, medicine, and social inequality, and as the acclaimed author of award-winning books, including The Social Life of DNA: Race, Reparations, and Reconciliation after the Genome (2016); Body and Soul: The Black Panther Party and the Fight against Medical Discrimination (2011); Genetics and the Unsettled Past: The Collision of DNA, Race, and History (2012; with Keith Wailoo and Catherine Lee); and Technicolor: Race, Technology, and Everyday Life (2001; with Thuy Linh Tu). Before joining the Biden Administration, Nelson was co-chair of the National Academy of Medicine Committee on Emerging Science, Technology, and Innovation and was a member of the National Academy of Engineering Committee on Responsible Computing Research. She served as a past president of the Social Science Research Council, an international research nonprofit, and was previously the inaugural Dean of Social Science at Columbia University. Dr. Nelson began her academic career on the faculty of Yale University, and there was recognized with the Poorvu Prize for interdisciplinary teaching excellence. Dr. Nelson is an elected member of the National Academy of Medicine, the American Academy of Arts and Sciences, the American Philosophical Society, the American Association for the Advancement of Science, and the American Academy of Political and Social Science.
Remarkable model performance makes news headlines and compelling demos, but these advances rarely translate to a lasting impact on real-world users. A common anti-pattern is overlooking the dynamic, complex, and unexpected ways humans interact with AI, which in turn limits the adoption and usage of AI in practical contexts. To address this, I argue that human-AI interaction should be considered a first-class object in designing AI applications.
In this talk, I present a few novel interactive systems that use AI to support complex real-life tasks. I discuss tensions and solutions in designing human-AI interaction, and critically reflect on my own research to share hard-earned design lessons. Factors such as user motivation, coordination between stakeholders, social dynamics, and user’s and AI’s adaptivity to each other often play a crucial role in determining the user experience of AI, even more so than model accuracy. My call to action is that we need to establish robust building blocks for “Interaction-Centric AI”—a systematic approach to designing and engineering human-AI interaction that complements and overcomes the limitations of model- and data-centric views.
Juho Kim
I will describe a training algorithm for deep neural networks that does not require the neurons to propagate derivatives or remember neural activities. The algorithm can learn multi-level representations of streaming sensory data on the fly without interrupting the processing of the input stream. The algorithm scales much better than reinforcement learning and would be much easier to implement in cortex than backpropagation.
Geoffrey Hinton
Rediet Abebe
NeurIPS has been in existence for more than 3 decades, each one marked by a dominant trend. The pioneering years saw the burgeoning of back-prop nets, the coming-of-age years blossomed with convex optimization, regularization, Bayesian methods, boosting, kernel methods, to name a few, and the junior years have been dominated by deep nets and big data. And now, recent analyses conclude that using ever bigger data and deeper networks is not a sustainable way of progressing. Meanwhile, other indicators show that Machine Learning is increasingly reliant upon good data and benchmarks, not only to train more powerful and/or more compact models, but also to soundly evaluate new ideas and to stress test models on their reliability, fairness, and protection against various attacks, including privacy attacks.
Simultaneously, in 2021, the NeurIPS Dataset and Benchmark track was launched and the Data-Centric AI initiative was born. This kickstarted the "data-centric era". It is gaining momentum in response to the new needs of data scientists who, admittedly, spend more time on understanding problems, designing experimental settings, and engineering datasets, than on designing and training ML models.
We will retrace the enormous collective efforts made by our community since the 1980's to share datasets and benchmarks, putting forward important milestones that led us to today's effervescence. We will pick a few hot topics that have raised controversy and have engendered novel thought-provoking contributions. Finally, we will highlight some of the most pressing issues that must be addressed by the community.
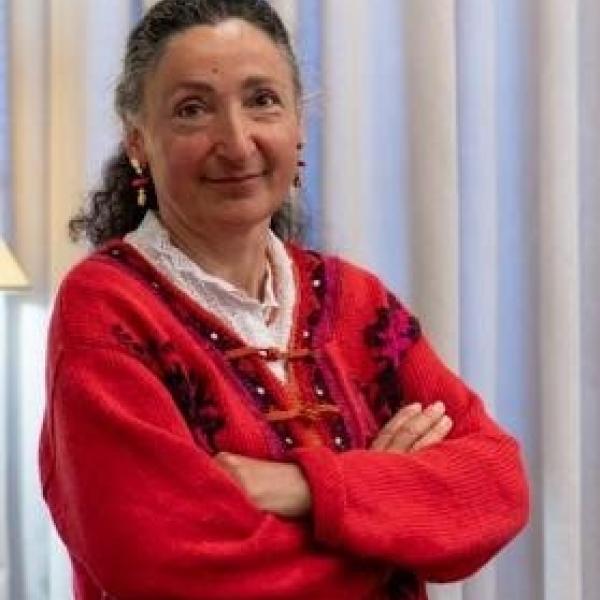
Isabelle Guyon
Isabelle Guyon recently joined Google Brain as a research scientist. She is also professor of artificial intelligence at Université Paris-Saclay (Orsay). Her areas of expertise include computer vision, bioinformatics, and power systems. She is best known for being a co-inventor of Support Vector Machines. Her recent interests are in automated machine learning, meta-learning, and data-centric AI. She has been a strong promoter of challenges and benchmarks, and is president of ChaLearn, a non-profit dedicated to organizing machine learning challenges. She is community lead of Codalab competitions, a challenge platform used both in academia and industry. She co-organized the “Challenges in Machine Learning Workshop” @ NeurIPS between 2014 and 2019, launched the "NeurIPS challenge track" in 2017 while she was general chair, and pushed the creation of the "NeurIPS datasets and benchmark track" in 2021, as a NeurIPS board member.